Data Scientists and others who wish to learn how to build and test robust prediction models for a range of problems.
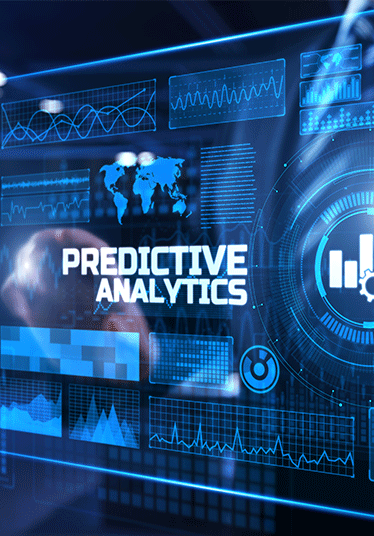
Predictive Analytics
Overview
Module Title | Predictive Analytics |
Subject Area | Data Science |
Credits | 5 |
NFQ | 9 |
EFQ | |
Start Date | 8th September 2025 |
Duration | 12 Weeks |
Time | N/A |
Mode of Delivery | Online |
Course Leader | TBC |
Fee | Full Fee: €900 *Subsidised Fee: €180 *80% Fee Subsidy for Eligible Learners (See below) |
Application Deadline | 29th August 2025 |
This module introduces students to simple statistical models with covariates, commonly known as regression models. Students will learn about the structure of such models, how to fit them using least squares, likelihood, and Bayesian methods, how to draw inferences, and how to check model fit. The module will use a variety of real-world examples to illustrate the use of regression models and cover both theoretical and practical considerations. Computer labs will make use of the R statistical package.
By the end of the module students should be able to:
- Interpret scatterplots for bivariate data.
- Define the correlation coefficient for bivariate data.
- Explain the interpretation of the correlation coefficient for bivariate data and perform statistical inference as appropriate.
- Calculate the correlation coefficient for bivariate data.
- Explain what is meant by response and explanatory variables.
- Derive the least squares estimates of the slope and intercept parameters in a simple linear regression model.
- Perform statistical inference on the slope parameter.
- Describe the use of measures of goodness of fit of a linear regression model.
- Use a fitted linear relationship to predict a mean response or an individual response with confidence limits
- Use residuals to check the suitability and validity of a linear regression model.
- State the multiple linear regression model (with several explanatory variables).
- Use appropriate software to fit a multiple linear regression model to a data set and interpret the output.
- Use measures of model fit to select an appropriate set of explanatory variables.
Topics covered:
- Matrix revision and Exploratory data analysis;
- Simple linear regression (SLR): properties of least squares estimates; t-tests; F-tests; Confidence intervals; Prediction intervals; Complete SLR analysis in the R statistical software;
- Multiple linear regression (MLR): properties of least squares estimates; t-tests; F-tests; Confidence intervals; Prediction intervals; Complete MLR analysis in the R statistical software;
- Categorical Predictors and Interactions
- Analysis of Variance
- Regression Diagnostics
- Variable Selection and Model Building
All the material is supplemented with its implementation in the R programming language which is rated 7th in IEEE list of top programming languages. The module will use a variety of real-world examples to illustrate the use of regression models and cover both theoretical and practical considerations.
You will become expert at building, interpreting, and comparing models for prediction. You will be able to create efficient and accurate prediction models and critique model fit to data.
- Weekly video lectures.
- Weekly labs covering materials implementation in R.
- Weekly problem sets.
Learning Requirements:
Students are expected to have taken previous statistics/data analytics modules covering the basics of probability and inference. The modules STAT40720 and STAT30280 are considered sufficient.
Assignment – 20%
Open book exam – 80%
Group/class feedback, post-assessment.
Prof Cert in Data Science.
Please note: Learners can avail of only one form of funding per application.
Micro-Credentials Learner Fee Subsidy-Human Capital Initiative Pillar 3
The HCI Pillar 3 Micro-credential Learner Fee Subsidy has been introduced to enable more learners to address critical skills gaps and engage with lifelong learning through micro-credentials. The HCI Pillar 3 Micro-credential Learner Fee Subsidy is funded by Higher Education Authority (HEA) and the Department of Further and Higher Education, Research, Innovation and Science.
HCI Micro-credential Learner Fee Subsidies are available on identified micro-credentials only and in fixed numbers from March 2024 until October 2025.
Please see Eligibility Criteria for further information.
On successful completion of this micro-credential, you will receive credits as per the European Credit and Transfer System. These credits are recognised by the awarding institution as credits aligned to learning completed at postgraduate level.
If you have any questions about this micro-credential, or would like to speak to a UCD staff member, please contact (opens in a new window)microcredentials@ucd.ie.